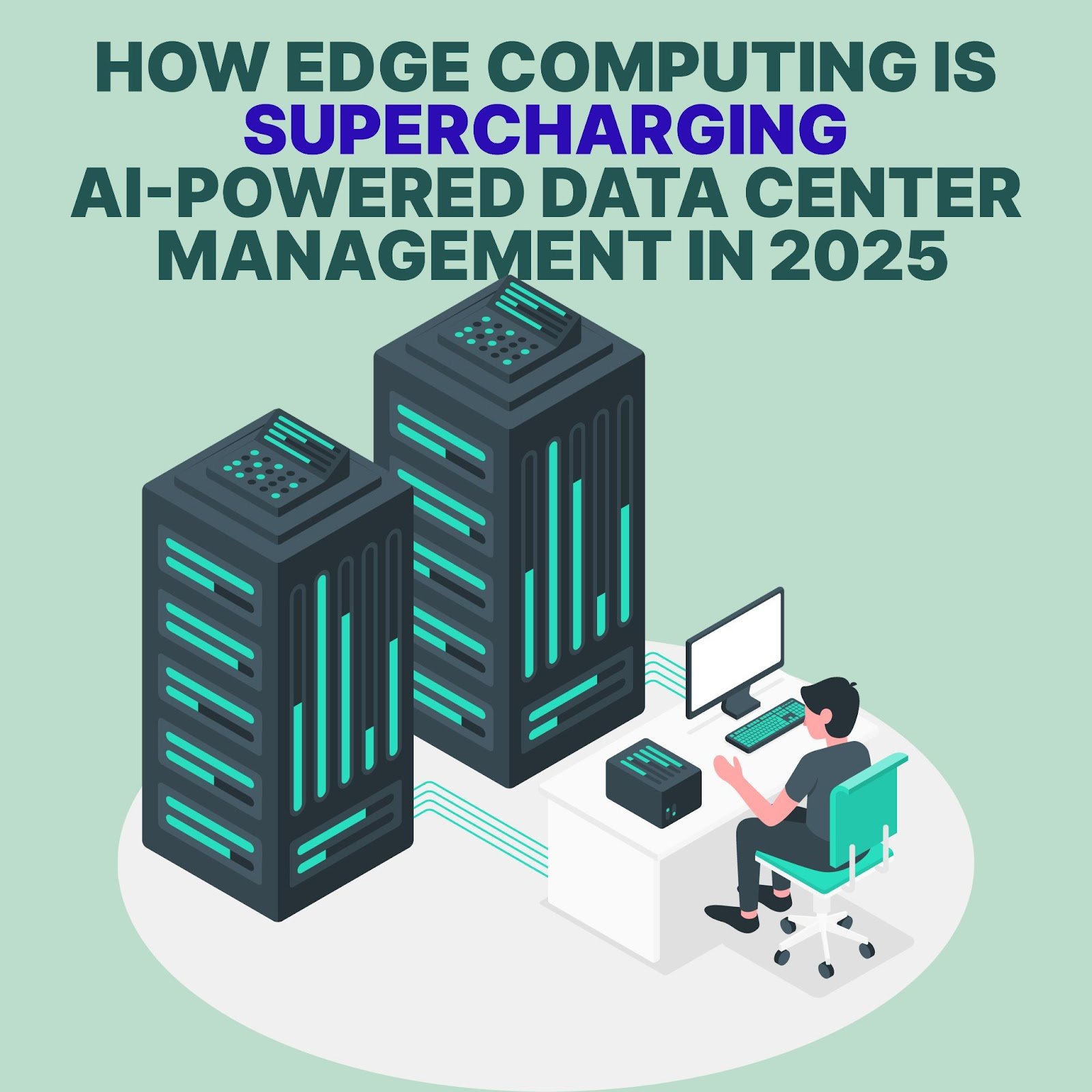
Before taliking about AI-Powered Data Center Management, let’s take a step back to when traditional data center management relies on centralized servers located miles away from end devices, introducing significant latency, bandwidth bottlenecks, and single points of failure. AI models often process raw data in these distant locations, delaying critical insights crucial for real-time decision-making.
Imagine a global retail chain during peak holiday season unable to detect stock shortages in real time because its AI analytics run hundreds of miles away. Envision an autonomous delivery drone hesitating mid-flight due to delayed obstacle recognition—milliseconds that could result in a lost package or damaged equipment.
Edge computing brings AI-Powered Data Center Management directly to the network’s edge, where data is generated. By deploying AI at edge nodes—micro data centers, smart gateways, and embedded devices—organizations meet AI data center requirements for low latency, real-time analytics, and resilient operations. Edge AI algorithms process video feeds, sensor readings, and user interactions locally, triggering immediate actions and upstream summaries only for aggregated insights.
Ready to future-proof your infrastructure? Contact Reboot Monkey today to explore our AI-Powered Data Center Management solutions and start your journey to edge intelligence.
The Core Concepts of Edge Computing
Edge computing represents a paradigm shift from centralized cloud processing to a distributed architecture. Rather than transmitting all raw data to distant data centers, edge nodes perform initial processing near the data source, reducing bandwidth use and cutting latency dramatically. This model aligns with AI data center requirements by preserving critical microseconds needed for inference.
What Is Edge Computing in Simple Terms?
In essence, edge computing moves compute resources—servers, AI accelerators, and storage—closer to sensors, cameras, and user devices. Instead of sending terabytes of raw video or telemetry across networks, edge nodes run AI models to extract actionable insights onsite. They then forward condensed results or anomalies to central systems, minimizing network congestion and ensuring faster response times.
Data Processing Closer to the Source
Local inference enables sub-50ms response times, meeting stringent AI data center requirements. Applications like real-time video analytics, predictive maintenance, and anomaly detection rely on edge AI to filter and pre-process data, sending only essential metadata or alerts upstream for long-term storage and batch training.
Use Cases from Retail to Robotics
- Retail: AI-powered shelf cameras continuously analyze product availability and shopper behavior. When stock runs low, the system generates automatic restocking alerts, reducing out-of-stock losses by up to 20%.
- Robotics: Autonomous robots in manufacturing facilities run miniature AI models on-device, recognizing parts and obstacles in under 10ms, which improves throughput and safety.
- Healthcare: Portable ultrasound and diagnostic devices execute AI-powered image analysis at the bedside, delivering instant insights while keeping sensitive patient data local.
Types of Edge Deployments
Edge computing encompasses multiple deployment tiers, each serving distinct use cases and environments.
Device Edge, Micro Data Centers, Metro Edge, Far Edge
- Device Edge: AI accelerators embedded in smartphones, sensors, and wearables perform on-device inference, enabling offline capabilities and preserving privacy.
- Micro Data Centers: These compact, containerized units house servers, storage, and cooling systems, often installed in telco central offices, factory floors, or retail backrooms.
- Metro Edge: Facilities in city hubs connect regional workloads via high-bandwidth fiber and 5G, supporting applications like smart traffic management and augmented reality.
- Far Edge: Remote installations—such as offshore oil rigs or rural renewable energy sites—process data on-site and use satellite or private LTE links to sync with core data centers periodically.
Examples in Autonomous Vehicles and Smart Grids
- Autonomous Vehicles: Edge nodes onboard vehicles process LIDAR, radar, and camera feeds in real time, executing obstacle detection and path planning within milliseconds.
- Smart Grids: Distributed edge controllers analyze sensor data from substations to balance supply and demand, preventing outages and optimizing energy distribution across the network.
Key Benefits and Limitations
Edge computing offers transformative gains but also introduces new operational complexities.
Lower Latency, Data Sovereignty, Cost vs Complexity
- Lower Latency: Edge processing reduces round-trip delays from over 100ms to under 20ms, critical for time-sensitive AI applications like autonomous systems and financial trading.
- Data Sovereignty: Keeping sensitive data within regional or organizational boundaries helps satisfy GDPR, HIPAA, and other local regulations.
- Cost vs Complexity: While local processing cuts bandwidth costs, it demands distributed orchestration, security enforcement, and higher operational expertise.
Edge AI Trade-offs
- Pros: Improved resilience, offline capabilities, and reduced central load.
- Cons: Fragmented infrastructure management, complex software updates, and increased security attack surface across dozens or hundreds of edge sites.
Next-Gen Green Data Center Technologies: Inside the Sustainable Infrastructure Revolution
AI-Powered Data Center Management Fundamentals
Managing distributed edge nodes requires robust AI-driven orchestration systems.
Intelligent Automation in Edge Operations
Auto-scaling algorithms allocate compute resources based on real-time load, while self-healing modules detect faulty nodes and reroute tasks automatically. Routine updates—security patches, model retraining, and configuration changes—deploy via AI-managed pipelines.
Use of Digital Twins
Digital twins replicate physical edge sites in virtual environments, simulating workload scenarios to optimize cooling, power distribution, and compute placement. AI analyzes these virtual models to proactively suggest hardware refreshes and capacity upgrades before performance degradation occurs.
Thermal Management with Predictive AI
Excess heat at edge sites accelerates hardware wear and increases energy costs. Predictive AI addresses this proactively.
Heatmaps, Airflow Optimization, Smart HVAC
Edge platforms generate 3D thermal heatmaps using sensor arrays. AI models then adjust fan speeds, vent orientations, and coolant flows in real time to maintain uniform temperatures.
Energy Efficiency Gains
By optimizing thermal management, organizations can slash HVAC power draw by up to 30%, translating to millions in annual OPEX savings and significant carbon footprint reduction.
Real-Time Monitoring with AI Dashboards
Visibility into edge operations is critical for uptime and performance.
Edge Telemetry, Fault Detection, Smart Alerts
AI dashboards ingather CPU, GPU, memory, and network metrics from all edge nodes. Machine learning algorithms detect anomalies—overheating, traffic spikes, or hardware failures—and trigger alerts or auto-remediation workflows.
Use of AI-Powered Data Center Management PPT Formats
Pre-built PPT templates visualize key metrics—latency trends, energy consumption, fault incidence—enabling stakeholders to quickly assess health and ROI of edge deployments.
Curious how tailored edge hardware can accelerate your AI goals? Request a customized infrastructure assessment from Reboot Monkey and discover the optimal hardware mix for your unique edge environment.
Next-Gen Infrastructure for Edge AI
Hardware advances are unlocking powerful AI capabilities at the edge.
Modular, Scalable Hardware for the Edge
Converged edge systems integrate GPUs, NPUs, and SSDs into ruggedized enclosures. Scalability modules let operators add AI accelerators or storage shelves on demand.
Hardware Lifecycle Considerations
AI-driven telemetry tracks component wear—CPU cycles, battery health, SSD endurance—and forecasts end-of-life, automating refresh plans to guarantee 99.99% availability.
Powering the Edge: Energy and Cooling
Reliable power and efficient cooling underpin every edge site’s operations.
Battery Backups, Liquid Cooling, Renewable Integration
Smart UPS systems use machine learning to predict battery degradation, scheduling replacements before failures. Liquid cooling solutions remove heat more efficiently in high-density racks, while integrated renewable microgrids (solar, wind) supplement grid power.
Remote Power Monitoring via AI
AI platforms continuously analyze UPS telemetry—charge cycles, temperature swings—to detect early warning signs of failure, triggering preventive maintenance or automated safe shutdown sequences.
Connectivity and Edge Networking Protocols
High-speed, resilient networks connect edge sites to central systems and each other.
5G, Wi-Fi 7, Private LTE, Low-Earth Orbit Satellite Links
5G and Wi-Fi 7 provide multi-gigabit connectivity in urban deployments. Private LTE offers licensed reliability in industrial settings. For remote far-edge sites, low-earth orbit satellites ensure periodic synchronization.
Redundancy and Resilience Strategies
AI-driven path selection balances traffic across multiple links. In case of a link failure, automated failover shifts workloads seamlessly to healthy connections, preserving real-time service levels.
Advanced AI Capabilities in Edge Data Centers
Edge data centers harness AI beyond basic orchestration.
AI for Load Balancing and Traffic Forecasting
Predictive models forecast resource demand—CPU, GPU, network—minutes in advance. This enables proactive scaling of edge nodes to prevent bottlenecks during peak loads.
Edge Routing Decisions in Milliseconds
Real-time AI algorithms analyze link latency and node health, rerouting traffic to underutilized nodes within sub-10ms windows, ensuring consistent performance.
China built hundreds of AI Data Centers To Catch The AI Boom | Reboot Monkey
Natural Language Interfaces for Data Center Admins
NLP makes edge management accessible to non-experts.
AI Copilots, Voice Command Systems, AI-Driven ITSM
Admins can speak natural queries—”Show CPU load for node Beta-3 over the last hour”—and receive interactive dashboards. AI copilots suggest remediation steps based on historical incidents.
Real-Time Documentation and Responses
AI chatbots generate incident tickets, draft root-cause analyses, and even update knowledge bases automatically.
Federated Learning at the Edge
Collaborative AI training without centralizing sensitive data.
AI Training Without Centralized Data
Each edge node trains a local model on its data, sending only gradient updates to a central aggregator. This approach meets strict privacy regulations and reduces network load.
Privacy, Security, and Distributed Model Training
Techniques like differential privacy and secure multi-party computation safeguard data during training aggregation.
Real-World Implementations and Case Studies
Concrete examples highlight edge AI’s impact.
Case Study: AI-Driven Efficiency in Data Centers
A major telecommunications provider deployed edge orchestration across 200 micro data centers. AI-driven load balancing cut average round-trip latency from 80ms to 20ms and reduced energy consumption by 25%, saving 3.2 million kWh annually.
Metrics Achieved: Latency Reduction, Energy Savings
- Latency: Decreased by 75%—from 80ms down to 20ms—improving user experience for real-time applications.
- Energy: Cut HVAC and compute power by 25%, equivalent to powering 2,000 homes for a year.
Industrial Edge: Smart Manufacturing & Logistics
Edge AI drives transformation in heavy industries.
Use of AI + Edge in Predictive Maintenance, Robotics
Edge nodes process vibration and temperature data from machinery, predicting failures up to two weeks in advance. This preemptive maintenance reduces downtime by 40%.
Example: Digital Twin Factories
Digital twin replicas simulate factory workflows, allowing AI to optimize robot pacing, conveyor speeds, and work schedules—boosting throughput by 15%.
Edge in Healthcare and Remote Diagnostics
Critical care benefits from immediate insights.
Real-Time Image Analysis, Patient Data Sovereignty
Edge-enabled MRI and CT scanners apply AI filters on-device, delivering diagnostic results in under a minute. By keeping patient data local, hospitals comply with jurisdictional privacy laws.
Edge AI’s Role in Diagnostics and Monitoring
Edge AI continuously monitors patient vitals—ECG, blood pressure, oxygen saturation—detecting anomalies in real time and issuing alerts to clinicians within seconds. In rural clinics, portable AI-enabled devices analyze samples and send summarized results upstream, reducing lab processing delays by up to 70%.
Discover Reboot Monkey‘s edge AI solutions in healthcare for HIPAA-compliant, real-time diagnostics!
AI at the Retail Edge
Retailers are leveraging edge AI to enhance customer experiences and optimize operations.
In-Store Analytics, Supply Chain Optimization
Smart shelves equipped with AI cameras analyze product placements and shopper interactions locally. Edge AI predicts restocking needs and optimal product placement, reducing stockouts by 25% and boosting sales. In warehouses, edge nodes track conveyor runs and package flows, optimizing pick-and-pack operations.
Real-Time Edge Processing with AI
On-site AI inference handles thousands of video frames per second, enabling dynamic pricing updates, personalized promotions on digital signage, and real-time checkout assistance. This localized processing slashes network costs and meets AI data center demand for instant responses.
Strategic Business Drivers
Edge AI initiatives are driven by competitive pressures, cost savings, and new revenue streams.
AI Data Center Companies Leading Edge Innovation
Leading AI data center companies—such as Company A (hybrid edge-core orchestration), Company B (ultra-compact micro data centers), and Company C (AI-managed microgrid integration)—are pioneering edge AI solutions. These firms offer turnkey platforms combining hardware, software, and managed services to accelerate deployments.
Areas of Specialization and Scale
Some companies focus on telco edge, optimizing for low-latency network functions. Others specialize in industrial IoT, integrating ruggedized hardware for harsh environments. Retail-focused providers deliver end-to-end solutions for in-store analytics and supply chain automation.
Growth Metrics for Edge AI Infrastructure
Market analysts project rapid adoption and investment in edge AI.
Market Size Forecasts and CAGR Stats
The global edge AI market is expected to reach $45 billion by 2028, growing at a 35% CAGR. Fueled by 5G rollouts and IoT proliferation, investments span across manufacturing, healthcare, retail, and telecommunications.
Regional Trends and Sector-Wise Analysis
APAC leads in 5G edge deployments, particularly in smart cities and autonomous transport projects. North America dominates micro data center installations in retail and healthcare, while Europe emphasizes data sovereignty and green edge initiatives, driven by stricter sustainability regulations.
Choosing Edge Locations Strategically
Selecting optimal edge locations maximizes performance and compliance.
Proximity to Data, User Density, Utility Access
Place edge nodes within urban population centers, near major fiber routes and power substations. For industrial sites, deploy next to manufacturing lines to minimize sensor-to-node latency. In retail, position nodes above store floors or in nearby regional centers.
Environmental and Political Stability Factors
Evaluate local infrastructure reliability—power grid uptime, cooling availability—and regulatory landscape. Regions with unstable utilities or ambiguous data laws pose higher risks and operational costs.
Compliance, Sustainability, and Risk Management
Edge AI must balance innovation with governance.
Data Governance and Edge Regulatory Compliance
Implement automated policy engines that enforce encryption-at-rest, role-based access, and data retention rules per region. Real-time compliance checks use AI to flag unencrypted sensitive data and generate audit reports.
Auditing Edge AI Operations
Continuous monitoring logs AI model inferences, data access, and network transactions. Smart analytics identify policy violations and suspicious behaviors, enabling rapid incident response.
The Future of Colocation Services in Shanghai and Major Chinese Tech Hubs | Reboot Monkey
Carbon-Neutral Edge Data Centers
Sustainability is critical for modern edge deployments.
AI-Powered ESG Metrics
AI dashboards track Scope 1 and 2 emissions, energy consumption patterns, and carbon offsets in real time. Predictive models optimize workload scheduling to align with renewable energy availability, maximizing green energy use.
Edge Use of Renewable Microgrids
On-site solar panels and small wind turbines feed into AI-managed battery systems. During peak solar production, workloads shift to solar-powered nodes, reducing reliance on diesel generators by over 80%.
Cybersecurity for AI Edge Deployments
Protecting distributed edge nodes requires advanced defenses.
AI-Powered Threat Detection
Edge firewalls employ machine learning to analyze packet flows and detect anomalies in encrypted traffic. Behavioral analytics profile device patterns and isolate compromised nodes automatically.
Edge-Specific Risks and Mitigation Strategies
Implement zero-trust segmentation, secure boot, and runtime integrity checks. Regular AI-driven penetration tests and automated firmware updates mitigate vulnerabilities across hundreds of edge locations.
Tools, Standards, and Deployment Models
Standardization accelerates integration and interoperability.
Open Standards for Edge AI Management
Projects like LF Edge, ETSI MEC, and Open Compute Project define APIs and hardware specifications that prevent vendor lock-in. Adopting these standards simplifies multi-vendor deployments and integration with existing data center management platforms.
Benefits of Standards-Based Deployments
Standards reduce integration time by up to 40%, lower procurement costs, and ensure long-term compatibility. They also foster vibrant ecosystems of tools and community support.
Emerging Software Tools and Platforms
Open-source frameworks and vendor-neutral layers streamline edge AI.
Open-Source AI Edge Frameworks (KubeEdge, NVIDIA Fleet Command)
KubeEdge extends Kubernetes to the edge, providing device management, service discovery, and data synchronization. NVIDIA Fleet Command orchestrates AI models across cloud and edge nodes, handling updates, monitoring, and scaling.
Vendor-Neutral Management Layers
Platforms like Open Horizon and Eclipse ioFog abstract hardware differences, enabling model portability and unified telemetry across heterogeneous edge environments.
From PoC to Full Deployment: A Roadmap
A staged approach ensures successful edge AI rollouts.
Step-by-Step Guide from Planning to Scale
- Assess: Identify use cases, define AI data center requirements, and evaluate regulatory constraints.
- Pilot: Deploy micro data centers in controlled environments; validate AI models and monitor edge performance.
- Optimize: Use AI analytics to adjust resource allocation, thermal settings, and network paths.
- Scale: Expand regionally, automate provisioning, and integrate with central management via AI orchestration.
Toolkits and Maturity Models
Frameworks like dfVertex, EdgeWorks, and IDC’s maturity models guide assessments across governance, security, and technology dimensions. They help benchmark capabilities and prioritize investments.
The Future of Edge + AI
Edge AI’s evolution continues with emerging compute paradigms.
Convergence of Edge AI with Quantum and Neuromorphic Computing
Quantum accelerators at edge sites will enable complex simulations—fluid dynamics for autonomous drones or rapid genome sequencing—within milliseconds. Neuromorphic chips mimic brain-like architectures, offering ultra-low-power inferencing for battery-constrained devices.
Long-Term Implications
These innovations will drive ubiquitous intelligence—from smart cities predicting traffic patterns to decentralized research networks tackling climate models—reshaping industries and daily life.
Autonomous Micro Data Centers
Self-managing edge nodes redefine infrastructure.
Fully AI-Managed, Zero-Touch Edge Sites
Autonomous micro data centers handle provisioning, capacity planning, and failure recovery without human intervention. AI agents monitor health metrics, deploy workloads, and order replacement parts automatically.
Implications for Remote Regions and Disaster Zones
Deployable in rugged containers, these sites provide resilient connectivity and compute in off-grid areas, supporting disaster response and humanitarian missions.
Human-Machine Collaboration in Edge Operations
Edge AI enhances workforce capabilities and safety.
AI Copilots, Augmented Workforce, XR Integration
Field technicians use AR headsets with AI overlays that identify components, display real-time telemetry, and guide repair steps. AI copilots answer queries and document procedures automatically.
Training and Human Factors
AI-driven training modules adapt to each technician’s skill level, offering simulations and just-in-time assistance to reduce errors and accelerate onboarding.
Conclusion
Edge computing is revolutionizing AI-Powered Data Center Management by delivering low-latency, secure, and sustainable operations across industries. From retail shelves to autonomous vehicles, from healthcare diagnostics to remote disaster sites, edge AI enables rapid insights and resilient performance. Organizations embracing edge-first strategies—integrating advanced hardware, AI orchestration, and robust compliance—will lead the next wave of decentralized intelligence.
Don’t wait—contact Reboot Monkey today to architect your AI-Powered Data Center Management roadmap and harness the full power of edge AI!
FAQs
What is AI-Powered Data Center Management?
AI-Powered Data Center Management uses AI to automate and optimize both edge and core operations for high efficiency.
How does Edge AI enhance AI-Powered Data Center Management?
By processing data locally on edge nodes, Edge AI cuts latency and bandwidth needs while feeding real-time insights upstream.
What are the key benefits of AI-Powered Data Center Management?
It delivers sub-20ms responses, predictive fault detection, and dynamic workload scaling at the edge.
Which industries benefit most from AI-Powered Data Center Management?
Retail, healthcare, telecom, and manufacturing leverage edge AI for instant analytics and resilient operations.
How do I get started with AI-Powered Data Center Management?
Assess your AI data center requirements, pilot edge use cases, then scale with an expert AI management partner.
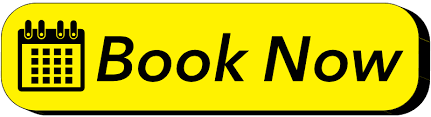
Leave a Reply
You must be logged in to post a comment.